i-PIΒΆ
i-PI is a universal force engine interface written in Python, designed to be used together with an ab-initio, machine-learned, or force-field based evaluation of the interactions between the atoms. You can see learn more about it on the ipi-code website, the documentation pages or the github repository.
Constant-temperature MD and thermostats
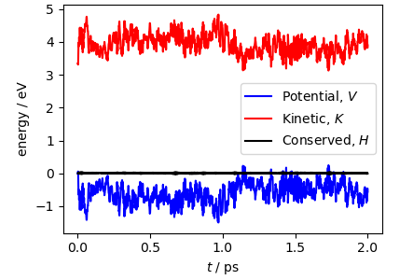
Path integral molecular dynamics
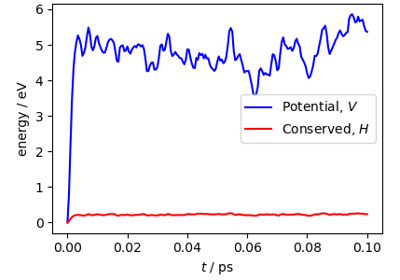
Path integral metadynamics
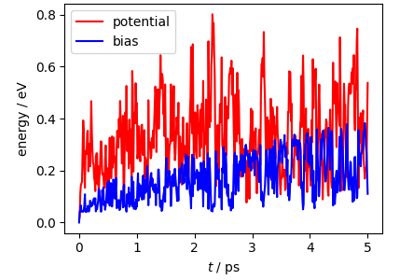
Quantum heat capacity of water
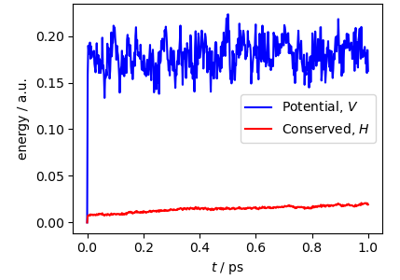
Multiple time stepping and ring-polymer contraction
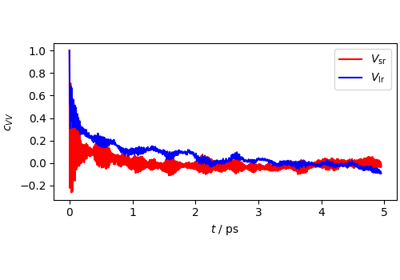
Atomistic Water Model for Molecular Dynamics
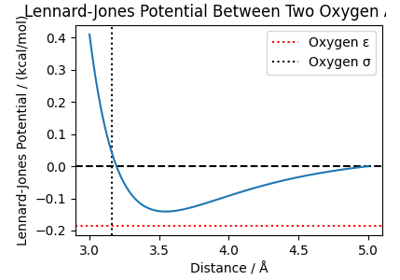
The PET-MAD universal potential
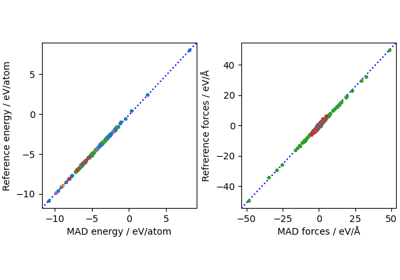