metatensor¶
Metatensor
is a library providing a cross-platform data interchange API
for atomistic simulation and beyond. It also powers
metatomic
– an API to define atomistic models that can be
used to run simulations using several different atomistic
simulation packages and metatrain
a set of tools to facilitate
training and evaluating ML models.
Atomistic Water Model for Molecular Dynamics
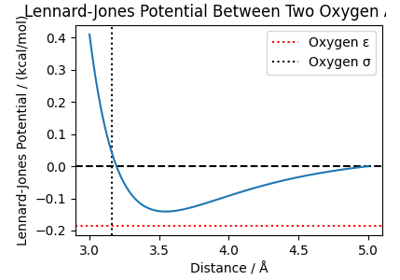
Equivariant linear model for polarizability
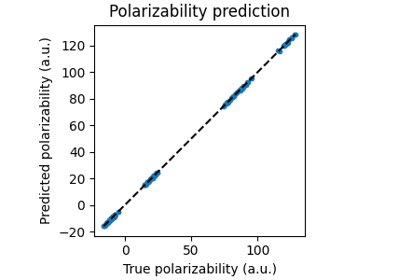
The PET-MAD universal potential
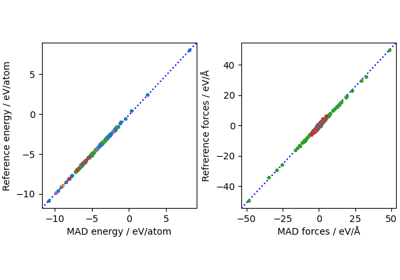